What is Data Analytics as a Service?
Introduction
Data Analytics is very diverse in the solutions it offers. It covers a range of activities that add value to businesses. It has secured a foothold in every industry that ever existed. Eventually carving a niche for itself known as Data Analytics as a Service (DAaaS)
DAaaS is an operating model platform where a service provider offers data analytics services that add value to a clients’ business. Companies can use DAaaS platforms to analyze patterns within the data using ready to use interface. Alternatively, companies can also outsource the whole data analytics task to the DAaaS providers.
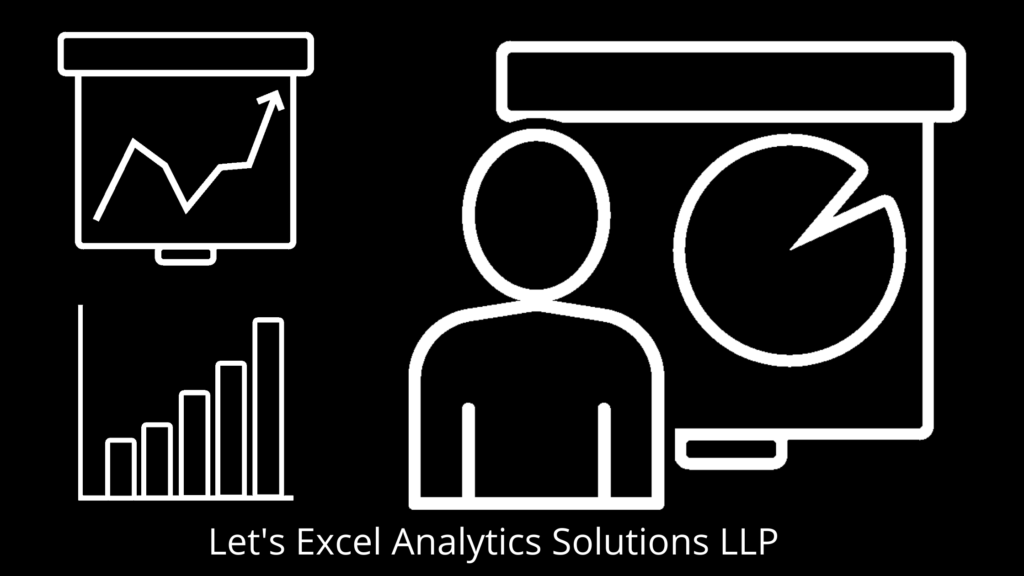
How does DAaaS Help Organizations?
Have you ever wondered how CEOs make big decisions? A potential game-changer that makes large companies trade high on the NYSE, NASDAQ, etc. A surprising statistic shows that organizations rely on intuition-based decision-making. High stake business decisions are made solely based on gut feelings and speculative explanations. However, there is an element of uncertainty associated with such decisions as long as that uncertainty is assessed. Data Analytics offers solutions on how data can be used to mitigate the associated risks and enable well-grounded decision-making.
Organizations collect data constantly on competitors, customers, and other factors that contribute to a business’s competitive advantage. This data helps them in strategic planning and decision-making. But the million-dollar question is whether organizations choose to build data analytics capabilities or outsource to Data Scientists with deep technical expertise. The answer to this question lies in the digital maturity of the organization. Most organizations prefer focusing on the core businesses rather than donning multiple hats at the same time. More and more organizations are turning to outsource their Data Science work to make most of their data. DAaaS furnishes the most relevant information extracted from data to help organizations make the best possible data-driven decisions.
Why Organizations Should Outsource Data Analytics
For many reasons, organizations, particularly start-ups, are turning to outsourced Data Analytics. Outsourcing has long been undertaken as a cost-cutting measure and is an integral part of advanced economies. Some of the main reasons why companies should opt for outsourcing Data Analytics include:
- Organizations can focus on core business.
- Outsourcing offers flexibility as the service can be availed only when it is required.
- Organizations don’t have to maintain a large infrastructure for data management.
- Organizations can advantage from high-end analytics services.
- Outsourcing has lower operational costs.
- It improves risk management.
What Can DAaaS Do for You?
Data Import
Data import is the first step towards building actionable insights. It helps organizations import data from their systems into the DAaaS platform. Data is an asset for organizations as it influences their strategic decision-making. Managing data is vitally important to ensure data is accurate, readily available, and usable by the organization.
Translate Data into Actionable Insights
Data is useful only when it is acted upon to derive useful insights that add value. Connecting and joining dots between data is important to put the facts and figures together. Data is nothing if the dots between them can’t be connected. The outcome of connecting and joining helps us answer one of the following bottom-line questions.
- What happened? Descriptive Analysis
- Why happened? Diagnostic Analysis
- What is likely to happen? Predictive Analysis
- What should be done? Prescriptive Analysis
Testing of ‘Trained Models’
Testing the accuracy of a model is the primary step in the implementation of the model. To test the accuracy of the model, data is divided into three subsets: Training Data, Testing Data, and Validation Data. A model is built on the training dataset that comprises a larger proportion of the data. Training data is subsequently run against test data to evaluate how the model will predict future outcomes. Validation data is used to check the accuracy and efficiency of the model. The validation dataset is usually the one not used in the development of the model.
Prediction and forecasting using ‘Trained Models’
Future events can be predicted using analytical models that has come to be known as predictive analytics. The analytical models are fit (also known as trained) using historical data. But such models constantly add data and eventually improve the accuracy of their prediction. Predictive analytics has been using advanced techniques like Machine Learning and Artificial Intelligence to improve the reliability and automation of the prediction.
Deploy Proven Analytical ‘Models’
Training a model is not quite as difficult as deploying a model. Deploying a model is the process of utilizing the trained model for the purpose it was developed for. It involves how the end-user interacts with the prediction of the model. The end-user can interact with the model using web services, a mobile application, and software. This is the phase that reaps the benefits of predictive modeling adding value to the business needs.
Conclusion
Data Analytics as a Service (DAaaS) companies enables access to high-tech resources without actually owning them. Organizations can reach out to DAaaS providers for their services only when it is required, eventually cutting huge costs on maintaining Data Analytics infrastructure and rare to find Data Scientists. This has enabled us to usher into a new world of the Gig Economy.
Let’s Excel Analytics Solutions LLP is a DAaaS company that offers a solution to all your Data Analytics problems.
Curious to know more?